Varela F. J. (1991) Whence perceptual meaning? A cartography of current ideas. In: Varela F. J. & Dupuy J. P. (eds.) Understanding origins: Contemporary ideas on the origin of life, mind and society. Kluwer, Boston: 235–263.
Available at https://cepa.info/2074 (free inscription, excellent knowledge base about enaction and alternative approaches to cognition).
Also published as a little book, in French: “Connaître – Les Sciences cognitives, tendances et perspectives” (Ed. Seuil, 1989), renamed “Invitation aux Sciences Cognitives” (Ed. Seuil, Point Science, 1996). But, sadly, it doesn’t seems that there is an English book version, so enjoy!
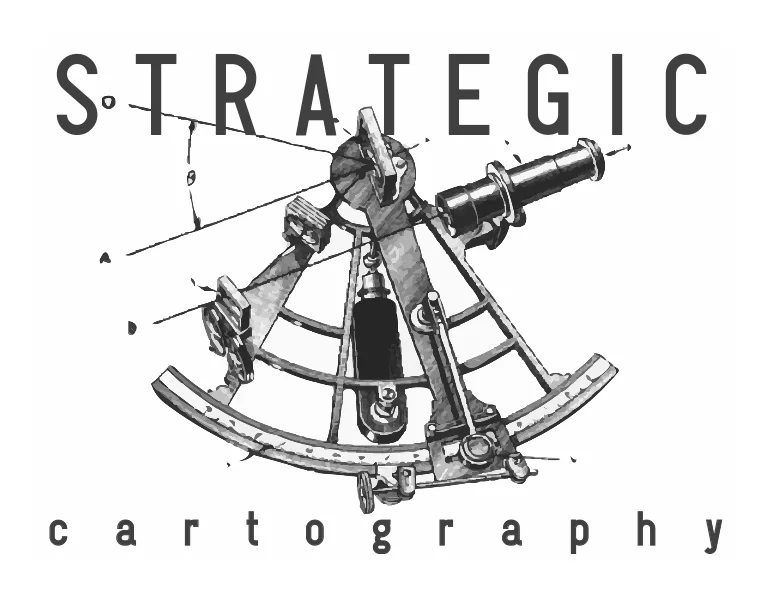
My personal comments and today’s enaction (optional)
This text has been on my my bedside table for years and even if the field has of course evolved in thirty years, most of it’s assertions and analysis are still valid today.
You will understand that the traditional scientific way of thinking is exemplified by the dominant, orthodox and intellectual “Cognitivism”, that the so-called “Deep Learning” techs are just one intermediary (“(neo)Connexionist”) way of seeing cognition, and that all these could be integrated into a comprehensive, embodied and situated approach: “Enaction“.
More, studying and practicing these 3 “ways of seeing”, and understanding their complementarity and useful respective contexts, is crucial and essential. Developing epistemological flexibility, an ability to navigate appropriately between these three points of view is the key to:
1. Potentially interpret any discourse about “cognition” or about “what is intelligence” but also to virtually conceptualize nearly any style of cognitive/”intelligent” system.
2. Understand and decode on what implicit and tacit preconceptions they rely (“metaphysical standpoint”), what Noopolitics & Technopolitics is at stake, what is missing.
3. And may be more importantly, to have beacons to reflect on your own thinking style.
>>> Trust me, if you ever had just one text to read (and study) about Cognitive Sciences in your life, it would be this one. And be patient if these views don’t “click” immediately in your mind, it’s absolutely normal, but don’t forget to come back to this essential synthetic reference. It will worth all the time you will put in it to familiarize yourself with them, and with you).
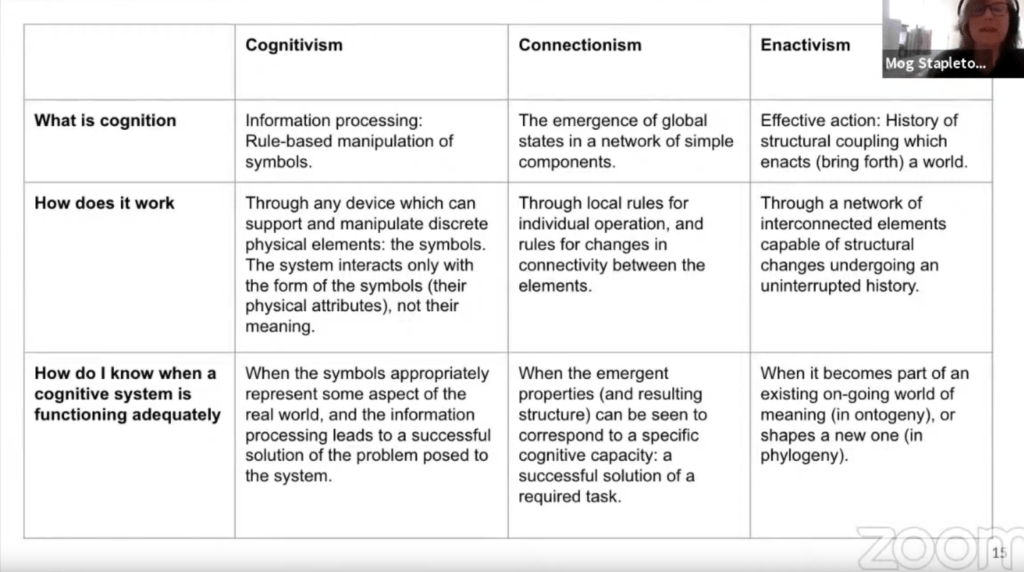
N.B.:
1. In this context it should be noted that here the term “symbol” doesn’t refer to it’s traditional and religious/spiritual/artistic sense (inner/outer synchronic meaningful evocation) but more to a kind of semiotic and relational sense as a physical “sign” (cf. Newell & Simon), devoid of embodied meaning.
2. One big absent of this overview is the Human & Social Sciences point of view, more covered by Dupuy (CREA). This is addressed more explicitly today by the “participatory sense-making” dimension (De Jaegher, Di Paolo) and the enactive ecological approach of psychology (Maiese), but also on the technical side by people like, for example, Havelange and Lenay (Compiègne School of enaction) in France. There is also others recent and exciting enactive approaches toward Philosophy (Thompson), Developmental & Neuro Biology (Ciaunica), Psychology (Renault), Phenomenology (Vörös, Bitbol, Depraz, Petitmengin), Physics (QuEn), Ecology (Weber), Human-machines interactions, Archeology, … and even a new “Irruption theory” (Froese), Art Philosophy & Aesthetic (Alva Noë) and love, just to name a few in my restricted field of view. Enactive scientists and thinkers are inherently multidisciplinary and powerful interface catalysts. Today’s Enaction is definitively alive and kicking!
But let’s dive into this masterpiece of a great and remarkable open-minded and visionary scientist:
°°°°~x§x-<@>
1. Introduction
Clarifications
This essay was written for the purpose of providing a minimal common ground for discussion. It is, of necessity, an ambitious attempt to give a concise account of the various current ideas on the origin of meaning in living and artificial systems in such a way that it is accessible to an interdisciplinary audience, and yet substantive enough to produce debate among the specialists. I apologize at the outset to both groups for passages that will seem irritatingly simple or too abstruse.
Also I have restrained myself to basic or ‘lower’ cognitive abilities, that is, issues closer to perception, motion, and simple learning. This is in contrast to ‘higher’ cognitive abilities, issues closer to language and reasoning.
This presentation cannot be neutral and my preferences are quite explicitly laid out in the text. In particular, in this essay I will argue that the kingpin of cognition is its capacity for bringing forth meaning: information is not pre-established as a given order, but regularities emerge from a co-determination of the cognitive activities themselves.
Outline
Cognitive Science (CS) is a little over 40 years old. It is not established as a mature science with a clear sense of direction and a large number of researchers constituting a community, as is the case of, say, atomic physics or molecular biology. Accordingly, the future development of CS is far from clear, but what has already been produced has had a profound impact, and this will continue to be the case. But progress in the field is based on daring conceptual bets (somewhat like trying to put a man on the moon … without knowing where the moon is). For the sake of concreteness, the federation of disciplines I take here as forming cognitive science today are neuroscience, artificial intelligence, cognitive psychology, linguistics, and epistemology. ❮︎Page 236❯︎
The main purpose of this background paper is to provide an X-ray picture of the current state of affairs of CS, in regards to perception and the origin of meaning. Now, like anybody who has ever examined a scientific discipline with any proximity, I have found the cognitive sciences to be a diversity of semi-compatible visions, and not a monolithic field. Further, as any social activity, it has poles of domination so that some of its participating voices acquire more force than others at different periods of time. This is strikingly so in the modern cognitive science revolution which was heavily influenced through some lines of research, particularly in the USA. My bias here is to emphasize diversity.
I will proceed in four stages which are conceptually and practically quite distinct. These four stages are the following:
- Stage 1: A glance at the foundational years (1943-1953);
- Stage 2: Symbols: The cognitivist paradigm;
- Stage 3: Emergence: alternatives to symbol manipulation;
- Stage 4: Enaction: alternatives to representations.
Through this four-tiered description and their articulations we will examine the basis of what is already established as a clear trend (Stages 1 and 2), and the fact that this established paradigm coexists with a wider spectrum of perspectives (Stages 3 and 4). This challenging heterodoxy has the potential for deep changes.
2. A glance at the foundational years
We start with a brief look into the roots of these ideas in the decade 1943-1953, so as to touch on the issues of direct relevance for us here. [Note 1] In fact, virtually all the themes in active debate today were already introduced in these formative years, evidence that they are deep and hard to tackle. The ‘founding fathers’ knew very well that their concerns amounted to a new science, and christened it with a new name: cybernetics. This name is not in current use any more, and many cognitive scientists today would not even recognize the family connection. This is not idle. It reflects the fact that to become established as a science, in its clear-cut cognitivist orientation (Stage 2 in this text), the future cognitive science had to sever itself from its roots; more complex and fuzzier, but also richer. This is often the case in the history of science: it is the price of passing from an exploratory stage to becoming a research program – from cloud to crystal.
The fruits of the cybernetics movement
The cybernetics phase of CS produced an amazing array of concrete results, apart from its long-term (often underground) influence. Some of these are:
- the use of mathematical logic to understand the operation of the nervous system;
- the invention of information processing machines (as digital computers), thus laying the basis for artificial intelligence;
- the establishment of the meta-discipline of system theory, which has had an imprint in many branches of science, such as engineering (system analysis, control theory), biology (regulatory physiology, ecology), social sciences (family therapy, structural anthropology management, urban studies), and economics (game theory);
- information theory as a statistical theory of signal and communication channels;
- the first examples of self-organizing systems.
The list is impressive: we tend to consider many of these notions and tools as an integral part of our lives. Yet they were all inexistent before this formative decade, and they were all produced by intense exchange among people of widely different backgrounds: a uniquely successful interdisciplinary effort.
Logic and the science of mind
The avowed intention of the cybernetics movement was to create a science of mind. To its readers, the mental phenomena had been for far too long in the hands of psychologists and philosophers, and they felt themselves called to express the processes underlying mental phenomena in explicit mechanisms and mathematical formalisms [Note 2]
One of the best illustrations of this mode of thinking (and its tangible consequences) was the seminal: ‘A logical calculus immanent in nervous activity [Note 3] (1943), paper by McCulloch and Pitts. Several major leaps were taken in this article. First, proposing that logic is the proper discipline with which to understand the brain and mental activity. Second, seeing the brain as a device which embodies logical principles in its component elements of neurons. Each neuron was seen as a threshold device being either active or inactive. Such simple neurons ❮︎Page 237❯︎ could then be connected to one another, their interconnections performing the role of logical operations so the entire brain could be regarded as a deductive machine.
These ideas were central for the invention of the digital computers. [Note 4] At that time, vacuum tubes were used to implement the McCulloch- Pitts neurons, where today we find silicon chips, but modern computers are still built on the same von Neumann architecture. This major technological breakthrough also laid the basis for the dominant approach to the scientific study of mind that was to crystallize in the next decade as the cognitivist paradigm.
The end of an era
There was, of course, a lot more to this creative decade. For instance, the debate about whether logic was indeed sufficient to understand the brain, because it neglected its distributed qualities. Alternative models and theories were put forth, which for the most part were to lay dormant until revived to constitute an important alternative for CS in the 1970s (Stage 3). By 1953, in contrast to their initial vitality and unity, the main actors of the cybernetics phase were distanced from each other and many died shortly thereafter. The idea of mind as logical calculation was to be continued.
3. Symbols: The cognitivists hypothesis
Enter the cognitivists
Just as 1943 was clearly the year in which the cybernetics phase was born, so was 1956 clearly the year which gave birth to the second phase of CS. During this year, at two meetings held at Cambridge and Dartmouth, new voices (like those of Herbert Simon, Noam Chomsky, Marvin Minsky and John McCarthy) put forth ideas which were to become the major guidelines for modern cognitive science. [Note 5]
The central intuition is that intelligence (including human intelligence) so resembles a computer in its essential characteristics that cognition can be defined as computations of symbolic representations. Clearly this orientation could not have emerged without the basis laid during the previous decade, and evoked in the previous section. The main difference is that one of the many original tentative idea is promoted here ❮︎Page 238❯︎ to full blown hypothesis, with a strong desire to set its boundaries apart from its broader, exploratory, and interdisciplinary roots where the social and biological sciences figured preeminently with all their multifarious complexity. Cognitivism [Note 6] is a convenient label for this large but well-delineated orientation, that has motivated many scientific and technological developments since 1956, in all the areas of cognitive science.
An outline of the doctrine
The cognitivist research program can be summarized as answers to the following questions:
Question # 1: What is cognition:
Answer: Information processing: Rule-based manipulation of symbols.
Question # 2: How does it work?
Answer: Through any device which can support and manipulate discrete physical elements: the symbols. The system interacts only with the form of the symbols (their physical attributes), not their meaning.
Question # 3: How do I know when such a cognitive system is functioning adequately?
Answer: When the symbols appropriately represent some aspect of the real world, and the information processing leads to a successful solution of the problem posed to the system.
Obviously the cognitivist program as outlined above did not come out ready-made, like Athena from the head of Zeus. We are presenting it with the benefits of 30 years of hindsight. However, not only has this bold research program become fully established, but even today is identified by many with cognitive science itself, although this is changing rapidly. Until very recently, only a few among its active participants, let alone in the public at large, were sensitive to its roots or its current challenges and alternatives. “The brain processes information from the outside world” is a household phrase understood by everybody. It is odd to treat statements such as this as problematic rather than obvious, and the ensuing conversation will immediately be labeled as being ❮︎Page 239❯︎ ‘philosophical’. This is a blindness in contemporary common sense introduced in our culture after the establishment of cognitivism.
What cognitivism has wrought: Artificial intelligence
The manifestations of cognitivism are nowhere more visible than in artificial intelligence, which is the literal construal of the cognitivist hypothesis. Over the years many interesting theoretical advances and technological applications have been made within this orientation: expert systems, robotics, image processing. These results have been widely publicized, and we need not insist on examples here.
Because of its wider implication, however, it is worth noting that AI and its cognitivist basis has reached a dramatic climax in Japan’s ICOT Fifth Generation Program. For the first time since the war there is a national plan concerting the efforts of industry, government, and universities. The core of this program – the rocket to be put on the moon by 1992 – is a cognitive device capable of understanding human language, and of writing its own programs when presented with a task by an untrained user. Not surprisingly, the heart of the ICOT program is the development of a series of interfaces of knowledge representation and problem solving based on PROLOG, a high level programming language for predicate logic. The ICOT program has triggered immediate responses from Europe and in the USA, and there is little question that this is a major commercial and engineering battlefield. However, what concerns us here is not whether the rocket will be built or not, but whether it points where the moon is. More about this latter.
Cognitive psychology
The cognitivist hypothesis finds its most literal construal in AI. Its complementary endeavor is the study of natural, biologically implemented cognitive systems, most especially man. Here, too, computationally characterizable representations have been the main explanatory tool. Mental representations are taken to be occurrences of a formal system, and the mind’s activity is what gives these representations their attitudinal color: beliefs, desires, plans, and so on. Here, therefore, unlike AI, we find an interest in what the natural cognitive systems are really like, and it is assumed that their cognitive representations are about something for the system, they are intentional. [Note 7]❮︎Page 240❯︎
A good example of this orientation of research is the following. Subjects were presented with geometric figures and asked to rotate them in their heads. They consistently reported that the difficulty of the task depended on the number of degrees of freedom in which the figure had to be rotated. That is, everything happens as though we have a mental space where figures are rotated like on a television screen. [Note 8] In due time these experiments produced an explicit theory postulating rules by which the mental space operates, similar to those used on computer displays operating on stored data. These researchers proposed that there is an interaction between language-like operations and picture-like operations, and together they generate our internal eye. [Note 9] This approach has generated an abundant literature, both for and against, [Note 10] and every level of the observations has been given alternative interpretations. However, the study of imagery is a perfect example of the way the cognitivist approach proceeds when studying mental phenomena.
Information processing in the brain
Another equally important effect of cognitivism is the way it has shaped current views about the brain. Over the years almost all of neurobiology (and its huge body of empirical evidence) has become permeated with the information-processing perspective. More often than not, the origins and assumptions of this perspective are not even questioned. [Note 11]
The best example of this approach is given by the celebrated studies on the visual cortex, where one can detect electrical responses from neurons when the animal is presented with a visual image. It was reported early on that it was possible to classify these cortical neurons as ‘feature’ detectors, responding to certain attributes of the object being presented: its orientation, contrast, velocity, color, and so on. [Note 12] In line with the cognitivist hypothesis, these results were seen as giving biological substance to the notion that the brain picks up visual information from the retina through the feature specific neurons in the cortex, and the information is then passed on to later stages in the brain for further processing (conceptual categorization, memory associations, and eventually action).
In its most extreme form, this view of the brain is expressed in Barlow’s [Note 13] grandmother cell doctrine, where there is a correspondence ❮︎Page 241❯︎ between concepts or percepts and neurons. (This is the AI equivalent of detectors and labeled lines.)
A brief outline of dissent
CS-as-cognitivism is a well-defined research program, complete with prestigious institutions, journals, applied technology and international commercial concerns. Most of the people who work within CS would subscribe – knowingly or unknowingly – to cognitivism or its close variants. After all, if one’s bread and butter consists in writing programs for knowledge representation, or finding neurons for well-defined tasks, how could it be otherwise? For our concerns here, it is important to draw attention to the depth of this social commitment from a large sector of the research community in CS. We now focus on the dissent, taking two basic forms:
- A critique of symbolic computations as the appropriate carrier for representations;
- A critique of adequacy of the notion of representations as the Archimedes’s point for CS.
4. Emergence: Alternatives to symbols
The roots of self-organization ideas
Alternatives to the towering dominance of logic as the main approach to CS had already been proposed and widely discussed during the formative decade. At the Macy Conferences, for example, it was argued that in actual brains there are no rules or central logical processor nor is information stored in precise addresses. Rather, brains seem to operate on the basis of massive interconnections, in a distributed form, so that their actual connectivity changes as a result of experience. In brief, they present a self-organizing capacity that is nowhere to be found in logic. In 1958 F. Rosenblatt built the Perceptron, a simple device with some capacity for recognition, purely on the basis of the changes of connectivity among neuron-like components; [Note 14] similarly, W. R. Ashby carried out the first study of the dynamics of very large systems with random interconnections, showing that they exhibit coherent global behaviors. [Note 15]❮︎Page 242❯︎
History would have it that these alternative views were bracketed out of the intellectual scene in favour of the computational ideas discussed above. It was only during the late 70s that an explosive rekindling of these ideas took place – after 30 years of preeminence of the cognitivist orthodoxy; what D. Dennett [Note 16] has called High Church Computationalism. Certainly one of the contributing factors for this renewed interest was the parallel rediscovery of self-organizational ideas in physics and non-linear mathematics.
Motivation to look for an alternative
The motivation to take a second look at self-organization was based on two widely acknowledged deficiencies of cognitivism. The first is that symbolic information processing is based on sequential rules, applied one at the time. This famous von Neumann bottleneck is a dramatic limitation when the task at hand requires large numbers of sequential operations (such as natural image analysis or weather forecasting). A continued search for parallel processing algorithms on classical architectures has met with little success because the entire computational philosophy runs precisely counter to it.
The second important limitation is that symbolic processing is localized: the loss of any part of the symbols or rules of the system implies a serious malfunction. In contrast a distributed operation is highly desirable, so that there is at least a relative equipotentiality and immunity to mutilations.
These two deviations from cognitivism can be phrased as the same: the architectures and mechanisms are far from biology. The most ordinary visual tasks, done even by tiny insects, are done faster than is physically possible when simulated in a sequential manner; the resiliency of the brain to damage without compromising all of its competence, has been known to neurobiologists for a long time.
What is emergence?
The above suggests that instead of focusing on symbols as a starting point, one could start with simple (non-cognitive) components which would connect to one another in dense ways. In this approach each component operates only in its local environment, but because of the network quality of the entire system, there is global cooperation which ❮︎Page 243❯︎ emerges spontaneously, when the states of all participating components reach a mutually satisfactory state, without the need for a central processing unit to guide the entire operation. [Note 17] This passage from local rules to global coherence is the heart of what used to be called self- organization during the foundations years. [Note 18] Today, different people prefer to speak of emergent or global properties, network dynamics, or even synergetics. Although there is no unified formal theory of emergent properties, the most obvious regional theory is that of attractors in dynamical systems theory. [Note 19] These are not the property of an individual components, but of the entire system, yet each component contributes to its emergence and characteristics.
A change of perspective concerning the brain
Recent work has produced some detailed evidence of how emergent properties are at the core of the brain’s operation. This is hardly surprising if one looks at the details of the brain’s anatomy. For example, although neurons in the visual cortex do have distinct responses to specific ‘features’ of the visual stimuli, as mentioned above, this is valid in an anesthetized animal with a highly simplified (internal and external) environment. When more normal sensory surroundings are allowed, and the animal is studied awake and behaving, it has been shown that and the stereotyped neuronal responses previously described become highly context sensitive. For example, there are distinct effects produced by bodily tilt [Note 20] or auditory stimulation. [Note 21] Further, the neuronal response characteristics depend directly on neurons localized far from their receptive fields.[Note 22]
Thus, it has become increasingly necessary to study neurons as members of large ensembles which are constantly disappearing and arising through their cooperative interactions, and where every neuron has multiple and changing responsiveness to visual stimulation, depending on context. Even at the most peripheral end of the visual system, the influences that the brain receives from the eye is met by more activity that descends from the cortex. It is by the encounter of these two ensembles of neuronal activity that a new coherent configuration emerges, depending on the match : mismatch between the sensory activity and the ‘internal’ setting at the cortex. [Note 23] In general, an individual neuron participates in many such global patterns and bears little significance when taken individually. ❮︎Page 244❯︎
Although these examples are taken from the domain of vision for the sake of contrast with the example of the previous section, several other detailed analysis have proliferated recently. [Note 24] We do not need to insist on this point further.
The (neo)connectionist strategy
The brain has been (once more) a main source of metaphors and ideas for other fields of CS in this alternative orientation. Instead of starting from abstract symbolic descriptions, one starts with a whole army of simple stupid components, which, appropriately connected, can have interesting global properties. These global properties are the ones that embody/express the cognitive capacities being sought.
The entire approach depends, then, on the introduction of the appropriate connections and this is usually done through a rule for gradual change of connections starting from a fairly arbitrary initial state. Several such rules are available today, but by far the most explored is Hebb’s Rule, whereby changes in connectivity in the brain could arise from the degree of coordinated activity between neurons: if two neurons tend to be active together, their connection is strengthened; otherwise it is diminished. Therefore the system’s connectivity becomes inseparable from its history of transformation, and related to the kind of task defined for the system. Since the real action happens at the level of the connections, the name (neo)connectionism has been proposed for this direction of research.’
One of the important factors for the explosive interests in this approach today was the introduction of some effective methods to follow network changes, most notably statistical measures which provide the system with a global ‘energy’ function that assures its convergence. [Note 26] For instance, take N simple neuron-like elements, connect them reciprocally, and provide them with a Hebb-type rule. Next present this system with a succession of (non-correlated) patterns at some of its nodes, and at each presentation let the system reorganize itself by rearranging its connections following its energy gradient. After the learning phase, when the system is presented again with one of these patterns, it recognizes it, in the sense that it falls into a unique attractor, and internal configuration that is said to represent the learned item. The recognition is possible provided the number of patterns presented is not larger than about 0.15N. Furthermore, the system ❮︎Page 245❯︎ performs a correct recognition even if the pattern is presented with added noise, or the system is partially mutilated. [Note 27]
Another important technique favored by some researchers is back- propagation [used today in so-called “Deep Learning”]: changes in neuronal connections inside the network (hidden units) are assigned so as to minimize the difference between the network’s response and what is expected of it, much like somebody trying to imitate an instructor. [Note 28] NetTalk, a celebrated recent example of this method, is a grapheme-phoneme conversion machine that works by being shown a few pages of English text in its learning phase. As a result, NetTalk can read out loud a new text, in what many listeners consider deficient but comprehensible English. [Note 29]
Connectionist models provide, with amazing grace, a working model for a number of basic cognitive capacities, such as rapid recognition, associative memory and categorical generalization. The current work with this orientation is justified on several counts. First, cognitivist AI and neuroscience had few convincing results to account for or reconstruct some of the cognitive performances just described. Second, these models are quite close to biological systems, and this means that one can work with a degree of integration between AI and neuroscience that was hitherto unthinkable Finally, the models are general enough to be applied, with little modification, to a variety of domains [cf. today’s LLMs/”ChatGPT”, Big Data, etc].
An outline of the doctrine
This alternative orientation – connectionist, emergent, self-organization, associationist, network dynamical – is young and diverse. Most of those who would enlist themselves as members hold widely divergent views on what CS is and on its future. Keeping this disclaimer in mind here are the alternative answers to the previous questions:
Question # 1: What is cognition?
Answer: The emergence of global states in a network of simple components.
Question # 2: How does it work?
Answer: Through local rules for individual operation, and rules for changes in connectivity between the elements.
Question # 3: How do I know when a cognitive system is functioning adequately? ❮︎Page 246❯︎
Answer: When the emergent properties (and resulting structure) can be seen to correspond to a specific cognitive capacity: a successful solution of a required task.
Exeunt the symbols
One of the most interesting aspects of this alternative approach to CS in that symbols, in their conventional sense, play no role. This entails a radical departure from a basic cognitivist principle: the physical structure of symbols, their form, is forever separated from what they stand for, their meaning. This separation between form and meaning was the master stroke that created the computational approach, but it also implies a weakness when addressing cognitive phenomena at a deeper level. How do symbols acquire their meaning? Whence this extra activity which is, by construction, not in the cognitive system?
In situations where the universe of possible items to be represented is constrained and clear-cut (such as when a computer is being programmed, or when an experiment is conducted with a set of predefined visual stimuli), the assignment of meaning is clear. Each discrete physical item within the cognitive system is made to correspond to an external item (its referential meaning), a mapping operation which the observer easily provides. Remove these constraints, and the form of the symbol is all that is left, and meaning becomes a ghost, as it would if we were to contemplate the bit patterns in a computer whose operating manual was lost.
In the connectionist approach, meaning is linked to the overall performance (say in recognition or learning). Hence, meaning relates to the global state of the system, and is not located in a particular symbols. The form/meaning distinction at the symbolic level disappears, and reappears in a different garb: the observer provides the correspondence between the system’s global state and the world it is supposed to handle. This, is, then, a radically different way of working with representations. We shall return to this issue below.
5. Linking symbols and emergence
At this stage the obvious question to consider is the relation between the symbolic and emergent views on the origin of simple cognitive properties. The obvious answer is that these two views should be ❮︎Page 247❯︎ complementary top-down and bottom-up approaches, or that they should be pragmatically adjoined in a some mixed mode, or simply used at different stages. A typical example of this move is to describe early vision in connectionist terms, say up to primary visual cortex, but to assume that at the inferotemporal cortex level, the description should be based on symbolic programs But the conceptual status of such synthesis is far from clear, and concrete examples are still lacking.
In my view the most interesting relation between emergent and symbolic descriptions is one of inclusion, that is, the view of symbols as a higher level of description of properties embedded in an underlying distributed system. The case of the so-called genetic code is paradigmatic, and I will use it here for concreteness. For many years biologists considered protein sequences as being instructions coded in DNA. However, it is clear that DNA triplets are capable of predictably specifying an aminoacid in a protein if and only if they are embedded in the cell’s metabolism, that is, in the midst of thousands of enzymatic regulations in a complex chemical network. It is only by the emergent regularities of such network as a whole that we can bracket out this metabolic background, and treat triplets as codes for aminoacids. In other words, the symbolic description is possible at another level of description. Clearly, it is possible to treat such symbolic regularities in their own right, but their status and interpretation is quite different than if taken at face value, with independence of the substratum from which they arise. [Note 30]
The example of genetic information can be transposed directly to the cognitive networks with which neuroscientists and connectionist deal. In fact, some researchers have recently expressed this point of view. [Note 31] In Smolensky’s “Harmony theory” [Note 32] for example, fragmentary atoms of ‘knowledge’ about electrical circuits linked by distributed statistical algorithms, yields a model of intuitive reasoning in this domain. The competence of this whole system can be described as doing inferences based on symbolic laws, but their performance sits at a different level and is never achieved by reference to a symbolic interpreter. This point is graphically portrayed in Figure 1.
Thus, a fruitful link between a less orthodox cognitivism, relaxed to emerge from parallel distributed processing provided by self-organizational approaches is a concrete possibility, especially in engineering- oriented AI. This potential complementation will undoubtedly produce visible results, and might well become the dominant trend for many years in CS. ❮︎Page 248❯︎
Figure 1: (a) A Punch cartoon that depicts succinctly the cognitivist hypothesis. To catch its prey, this kingfisher has, in its brain, a representation of Snell’s law of refraction. (b) Another reading of the cartoon to indicate how the symbolic levels can be seen as arising from the underlying network. ❮︎Page 249❯︎
This move is, of course, inadmissible from a strict or orthodox cognitivist position. [Note 33] Among the many issues that change from the emergence viewpoint, two of them are worth underlining here. First, the question of the origin of a symbol and its signification (i.e. why does ATI code for alanine?) has at least a clear way to approach it. Second, any symbolic level becomes highly dependent on the underlying network’s properties and peculiarities, and bound to is history. A purely procedural account of cognition, independent of its embodiments and history, is, therefore, seriously questioned. These two issues take us straight into our last stage.
6. Enaction: Alternatives to representations
Further grounds for dissatisfaction
It is tempting to stop in an analysis of today’s CS with just the two approaches already discussed. But this would be inadequate, since in both orientations (and hence some future synthesis) some essential dimensions of cognition would be still missing. We need to keep in mind a larger horizon for CS, born from a deeper dissatisfaction than the search for alternatives to symbols, and closer to the very foundation of representational systems. Hopefully this orientation, enjoying today some breathing space, will not suffer the same fate as that of earlier self-organization ideas, left to be rediscovered after 30 years.
Insisting on common sense
The central dissatisfaction of what we here call the enactive alternative is simply the complete absence of common sense in the definition of cognition so far. Both in cognitivism (by its very basis) and in present day connectionism (by the way it is practiced), it is still the case that the criteria for cognition is a successful representation of an external world which is pre-given, usually as a problem solving situation. However, our knowledge activity in everyday life reveals that this view of cognition is too incomplete. Precisely the greatest ability of all living cognition is, within broad limits, to pose the relevant issues to be addressed at each moment of our life. They are not pre-given, but enacted or brought forth from a background, and what counts as relevant is what our common sense sanctions as such, always in a contextual way. ❮︎Page 250❯︎
This is a critique of the use of the notion of representation as the core of CS, since only if there is a pre-given world can it be represented. If the world we live in is brought forth rather than pre-given, the notion of representation cannot have a central role any longer. The depth of the assumptions we are touching here should not be underestimated, since our rationalist tradition as a whole has favored (with variants of course) the understanding of knowledge as a mirror of nature. It is only in the work of some continental thinkers (most notably M. Heidegger, M. Merleau-Ponty and M. Foucault) that the explicit critique of representations, and the enactive dimension of understanding has begun. These hermeneutical themes were first introduced as the discipline of the interpretation of ancient texts, but has now been extended to denote the entire phenomenon of interpretation understood as the activity of enactment or bringing forth, to which we are alluding. [Note 34] Since we are concerned here with the dominance of usage, instead of with representations, it is appropriate to call this alternative approach to CS enactive. [Note 35]
In recent years, however, a few researchers within CS have put forth concrete proposals, taking this critique from the philosophical level into the laboratory and into specific work in AI. This is a more radical departure from CS than the preceding one, and one that goes beyond the themes discussed during the formative period. At the same time, it naturally incorporates the ideas and methods developed within the connectionist context, as we shall presently see.
The problem with problem solving
The assumption in CS has all along been that the world can be divided into region of discrete elements and tasks to which the cognitive system addresses itself, acting within a given ‘domain’ of problems: vision, language, movement. Although it is relatively easy to define all possible states in the ‘domain’ of the game of chess, it has proven less productive to carry this approach over into, say, the ‘domain’ of mobile robots. Of course, here too one can single out discrete items (such as steel frames, wheels and windows in a car assembly). But it is also clear that while the chess world ends neatly at some point, the world of movement amongst objects does not. It requires our continuous use of common sense to configure our world of objects.
In fact, what is interesting about common sense is that it cannot be ❮︎Page 251❯︎ packaged into knowledge at all, since it is rather a readiness-to-hand or know-how based on lived experience and a vast number of cases, which entails an embodied history. A careful examination of skill acquisition, for example, seems to confirm this point. [Note 36] A lived, natural world does not have sharp boundaries, and thus we expect a symbolic representation with rules, to be unable to capture common-sense understanding. In fact, it is fair to say that by the 1970s, after two decades of humblingly slow progress, it dawned on many workers in CS that even the simplest cognitive action requires a seemingly infinite amount of knowledge, which we take for granted (in fact it is so obvious as to be invisible), but which must be spoon-fed to the computer. The cognitivist hope for a general problem-solver in the early 60s, had to be shrunk down to local knowledge domains with well-posed problems to be solved, where the programmer could project onto the machine as much of his/her own background knowledge as was practicable. Similarly, the commonly practiced connectionist strategy depends on restricting the space of possible attractors by means of assumptions about known properties of the world which are incorporated as additional constraints for regularization, [Note 37] or, more recently, in back propagation methods as a perfect model to be imitated. In both instances, the unmanageable ambiguity of background common sense is left at the periphery of the inquiry, hoping that it will be clarified in due time. [Note 38]
Such acknowledged concerns have a well-developed philosophical counterpart. Phenomenologists of the continental tradition have produced detailed discussions as to why knowledge is a matter of being in a world which is inseparable from our bodies, our language and social history. [Note 39] It is an ongoing interpretation which cannot be adequately captured as a set of rules and assumptions since it is a matter of action and history, an understanding picked up by imitation and by becoming a member of an understanding which is already there. Furthermore, we cannot stand outside the world in which we find ourselves, to consider how its contents match their representations of it: we are always already immersed in it. Positing rules as mental activity is factoring out the very hinge upon which the living quality of cognition arises. It can only be done within a very limited context where almost everything is left constant, a pervasive ceteris paribus condition. Context and common sense are not residual artifacts that can be progressively eliminated by the discovery of more sophisticated rules. They are in fact the very essence of creative cognition. ❮︎Page 252❯︎
If this critique is correct, even to some limited degree, progress in understanding cognition as it functions normally (and not exclusively in highly constrained environments) will not be forthcoming unless we are to start from another basis than a domain out-there to be represented.
Exeunt the representations
The real challenge posed to CS by this orientation is, then, that it brings into question the most entrenched assumption of our scientific tradition altogether: that the world as we experience it is independent of the knower. Instead, if we are forced to conclude that cognition cannot be properly understood without common sense, and this is none other than our bodily and social history, the inevitable conclusion is that knower and known, subject and object, stand in relation to each other as mutual specification: they arise together.
Consider the case of vision: which came first, the world or the image? The answer of vision research (both cognitivist and connectionist) is unambiguously given by the names of the tasks investigated: to ‘recover shape from shading’ or ‘depth from motion’, or ‘color from varying illuminants’. This we may call the chicken extreme:
Chicken position: The world out-there has fixed laws, it precedes the image that it casts on the cognitive system, whose task is to capture it appropriately (whether in symbols or in emergent states).
Now, notice how very reasonable this sounds, and how difficult it seems to imagine that it could be otherwise. We tend to think that the only alternative is the egg position:
Egg position: The cognitive system creates its own world, and all its apparent solidity is the primary reflection of the internal laws of the organism.
The enactive orientation proposes that we take a middle way, [Note 40] moving beyond these two extremes by realizing that (as farmers know) egg and chicken define each other, they are co-relative. It is the ongoing process of living which has shaped our world in the back-and-forth between what we describe as external constraints from our perceptual perspective and the internally generated activity. The origins of this process are for ever lost, and our world is for all practical purpose stable (… except when it breaks down). But this apparent stability need ❮︎Page 253❯︎ not obscure a search for the mechanisms that brought them forth. It is this emphasis on co-determination (beyond chicken and egg) which marks the difference between the enactive viewpoint and any form of constructivism [Note 41] or biological neo-Kantism. [Note 42] This is important to keep in mind, since the more or less realist philosophy that pervades cognitive science will tend to assume that anybody who questions representations must ipso facto be in the antipodal position where the spectre of solipsism also lives.
Color and smell as examples
The preceding considerations are usually made in the realm of language and human communications, and it would seem that for the more immediate perceptual world they would not be relevant. But the whole point is that enaction applies at all levels. Thus examining perception at this light is important.
Consider the world of colors that we perceive every day. It is normally assumed that color is an attribute of the wavelength of reflected light from objects that we pick it up and process it as relevant information. In fact, as has now been extensively documented, the perceived color of an object is largely independent of the incoming wavelength.’“ Instead, there is a complex (and only partially understood) process of cooperative comparison between multiple neuronal ensembles in the brain, which specifies the color of an object according to the global state it reaches: a perceptual chromatic space is specified.”
Now, clearly these mechanism are consistent with what we describe as illumination constraints (reflectance, object discontinuity, and so on) but they are not a logical consequence of them. The cooperative neuronal operations underlying our perception of color, have resulted from the long biology evolution of the primate group. Their effects are so pervasive to our life that it is tempting to assume that colors, as we see them, is the way the world is. But this conclusion is tempered if we remember that other species have evolved different chromatic worlds by performing different cooperative neuronal operations from their sensory organs. For example, the pigeon’s chromatic space is apparently tetrachromatic (requires four primary colors), in contrast to us trichromats (where only three primary colors suffice). [Note 45] This is not a merely expansion in diversity within the same spectrum, but an entirely ❮︎Page 254❯︎ new dimension which brings forth a chromatic world as incommensurable to ours as ours is to a daltonic person. Color here appears not as a correlate of world properties, but as regularities which are co-defined with a particular mode of being.
What can be said is that our chromatic world is viable: it is effective since we have continued our biological lineage. The vastly different histories of structural coupling of birds, insects, and primates have brought forth a world of relevance for each inseparable from their living. All that is required is that each path taken is viable, i.e. be an uninterrupted series of structural changes. The neuronal mechanisms underlying color are not the solution to a ‘problem’ (picking up the correct chromatic properties of objects), but the arising together of color perception and what one can then describe as chromatic attributes in the world inhabited.
Another perceptual dimension where these ideas can be seen at play is olfaction, not due to the comparative span provided by phylogeny, but due to novel electrophysiological techniques. Over many years of work, Freeman” has managed to insert an array of electrodes into the olfactory bulk of a rabbit so that a small portion of the global activity can be measured while the animal behaves freely. It was found that there is no clear pattern of global activity in the bulb unless the animal is exposed to one specific odor several times. Further, such emerging patterns seem to be created out of a background of incoherent activity into a coherent attractor. As in the case of color, smell reveals itself not as a passive mapping of external traits, but as the creative dimensioning of meaning on the basis of history. [Note 47]
In this light, then, the brain’s operation is centrally concerned with the constant enactment of worlds through the history of viable lineages; an organ laying down worlds, rather than mirroring.
An outline of the doctrine
The basic notion, then, is that cognitive capacities are inextricably linked to a history that is lived, much like a path that does not exist but is laid down in walking. Consequently, the view of cognition is not that of solving problems through representations, but as a creative bringing forth of a world where the only required condition is that it is effective action: it permits the continued integrity of the system involved. [Note 48] ❮︎Page 255❯︎
Question # 1: What is cognition?
Answer: Effective action: History of structural coupling which enacts (bring forth) a world.
Question # 2: How does it work?
Answer: Through a network of interconnected elements capable of structural changes undergoing an uninterrupted history.
Question # 3: How do I know when a cognitive system is functioning adequately?
Answer: When it becomes part of an existing on-going world of meaning (in ontogeny), or shapes a new one (in phylogeny).
It should be noted that two notions surface in these answers that are usually absent from considerations in CS. One is that, since representations no longer play a central role, intelligence has shifted from being the capacity to solve a problem to the capacity to enter into a shared world. The second is that what takes the place of task-oriented design is an evolutionary process. Bluntly stated, just as much as connectionism grew out of cognitivism inspired by a closer contact with the brain, the enactive orientation takes a further step in the same direction to encompass the temporality of living either in ontogeny and phylogeny.
Working without representations
Seeking alternatives to representation to study cognitive phenomena (and this is, admittedly, a vague umbrella, much as connectionism is) attracts a relatively small group of people in diverse fields. Further, as I shall argue in the next section, many of the tools of traditional connectionist perspective can be re-formulated in this context, so the divisory lines are much less sharp here than they were between the symbolic and connectionism orientations.
It is clear that an enactive strategy for AI is feasible only if one is prepared to relax the constraints of a specific problem solving performance. This is the spirit, for example, of the so-called classifier systems, [Note 49] conceived to confront an undefined environment which it has to shape into significance. More generally, simulations of prolonged histories of coupling with various evolutionary strategies permit to ❮︎Page 256❯︎ discover trends wherein cognitive performances arise [Note 50] But these new perspectives for research are only at their earliest beginnings.
7. Linking emergence and enaction
The link between emergence and enaction depends on changing one’s reading of what a distributed system can do. If one emphasizes how a historical process leads to emergent regularities without a fixed final constraint, one recovers the more open-ended biological condition. If one emphasizes, instead, how a given network will acquire a very specific capacity in a very definite domain (i.e. NetTalk), then representations are back in, and we have the more usual take on connectionist models. However, the first interpretation also entails a whole new different perspective on what cognition is, as outlined in the previous section.
Thus the road taken is strongly dependent on the degree of interest to stay closer to biological reality, and further away from a pragmatic-engineering considerations. Of course, defining a fixed domain within which a connectionist system can function is possible, but it obscures the deeper issues about origin so central to the enactive viewpoint.
Consider for example Smolensky’s Harmony theory. His viewpoint of sub-symbolic computation as a model for intuition seems eminently in line with an enactive perspective, which is why it can serve as the best case to consider here for contrast. However, even Harmony theory is evaluated in reference to an unviolated level of environmental reality: exogenous features matching given features of the world, and endogenous activity which acquire through experience a state of abstract meaning that “optimally encode environmental regularity.” The hope is to find endogenous activity which corresponds to an “optimality characterization” of the surroundings. [Note 51] The enactive perspective would require taking this kind of cognitive system into a situation where endogenous and exogenous are mutually definitory through a prolonged history requiring only a viable coupling, and eschewing any form of optimal fitness. [Note 52]
Granted, from the standpoint of a pragmatically oriented AI, having as objective the production of a system that works in some domain in short delay, this orientation seems pointless. My argument is that cognitive properties emerged in living systems without such optimality considerations. They result from histories of viable compensations that ❮︎Page 257❯︎ create regularities, but it is far from obvious that they can be said to correspond to some unique referent.
Thus, there is a tension between the two parallel worlds of research, where the choice for or against the enactive critique is taken according to all the complexities of a conceptual shift, and the technological world, where the straight-jacket of immediate applicability sets the limits on how far it is able to extend itself. It seems to me that this tension will probably be resolved by a widening gap between the technological and the scientific components of CS. [Note 53]
8. Conclusion: Embodied knowledge
We started from the hard-core of CS and moved towards what might be considered its periphery, that is, the consideration of surrounding context, and effects of the biological and cultural history on cognition and action. Of course, those who hold on to representations as a key idea, see these concerns as only temporarily outside of the more precise realm of problem-solving orientation that seems more accessible; others go as far as to take the position that such ‘fuzzy’ and ‘philosophical’ aspects should not even enter into a proper cognitive science.
Some contrasts that provide these tensions may be stated in the following table.
From: | Towards: |
task-specific | creative |
problem solving | problem definition |
abstract, symbolic | history, body bound |
universal | context sensitive |
centralized | distributed |
sequential, hierarchical | parallel |
world pre-given | world brought forth |
representation | effective action |
implementation by design | implementation by evolutionary strategies |
abstract | embodied |
As a visual summary of this presentation I have outlined the three main directions discussed here in a polar map of Figure 2. My view is ❮︎Page 258❯︎ that these three successive waves to understand basic cognition and its origin relate to each other by successive imbrication, as Chinese boxes. In the centripetal direction, one goes from emergence to symbolic by bracketing the base from which symbols emerge, and working with symbols at face value. Or one can go from enaction to a standard connectionist view by assuming given regularities of the domain where the system operates (i.e. a fitness function in a domain). The centrifugal direction is a progressive bracketing of what seems stable and regular, to consider where such regularities could have come from, up to and including perceptual dimensions of our human world.
Figure 2: A polar map of STC, having the cognitivist paradigm at the center and the alternative themes as fringe, both touching the double-edged body of connectionist ideas. Along the disciplinary radii, some names of workers in each representative area.
It is clear that each one of these approaches, as levels of descriptions, are useful in their own context. However, if our task is to understand ❮︎Page 259❯︎ the origin of perception and cognition as we find them in our actual lived history, I think that the correct level of explanation is the most inclusive outer rim of the map. Further, for an AI where machines are intelligent in the sense of growing into a common sense with human beings as animals can do, I can see no other route but to bring them up through a process of evolutionary transformations as suggested in the enactive perspective. [Note 54] How fertile, how difficult, or how impossible this will prove to be, is anybody’s guess.
My preferences have been quite explicitly laid out in the text. In particular, in this essay I have argue that, if the kingpin of cognition is its capacity for bringing forth meaning, then information is not pre-established as a given order, but it amounts to regularities that emerge from the cognitive activities themselves. It is this re-framing that has multiple ethical consequences, which should be evident by now. As pointed out in the last item of our table above, to the extent that we move from an abstract to a fully embodied view of knowledge, facts and values become inseparable. To know is to evaluate through our living, in a creative circularity.
°°°°~x§x-<@>
To go further, check out this part with the summary table and links to a recent video presentation and discussion of this essential text, and others resources about enaction.
And also this synthetic table representing the three historical trends and bifurcations of the Cybernetics movement / modelling style, by Stuart Umpleby.
1. First bifurcation between so called “AI” (founded) and Second-Cybernetics (neglected), also called here “Biological cybernetics”, leading to the Enactive movement.
2. A second bifurcation of the Second-cybernetics between Enactive natural and cognitives sciences, and more social developments (Umpleby, Luhmann, etc).
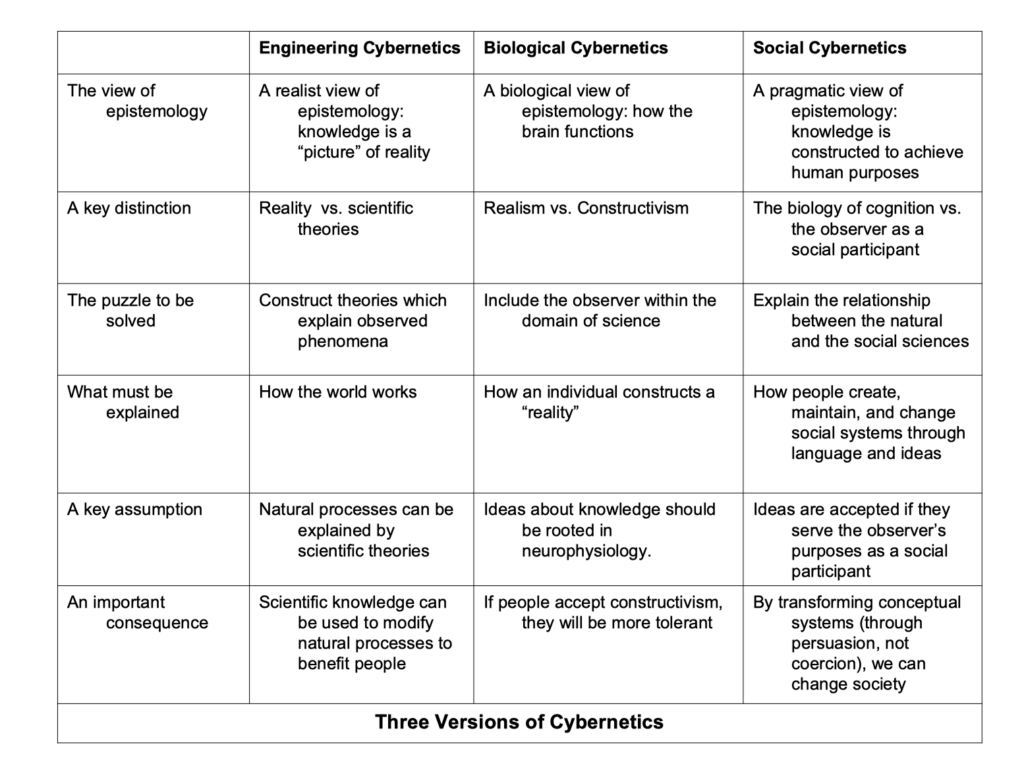
Endnotes
This Section owes much to our recent collective work on the neglected history of early cybernetics, self-organization, and cognition, published as Cahiers du CREA N’s 7-9. The only other useful source is S. Heims, John von Neumann and Norbert Wiener, MIT Press, 1980. The recent book by H. Gardner, The Mind’s New Science: A History of the Cognitive Revolution, Basic Books, 1985, discusses this period only in a superficial way.
The best sources here are the oft-cited Macy Conferences, published as Cybernetics- Circular causal and feedback Mechanisms in Biological and Social Systems, Josiah Macy Jr. Foundation, New York, 5 volumes.
Bulletin of Mathematical Biophysics, 5, 1943. Reprinted in W. McCulloch, Embodiments of Mind, MIT Press, 1965.
For an interesting perspective about this historical/conceptual moment see also A. Hodges, Alan Turing: The Enigma of Intelligence, Touchstone, New York, 1984.
See H. Gardner, op. cit., Chapter 5 for this period.
This designation is justified in J. Haugland (Ed.), Mind Design, MIT Press, 1981. Other designations used are: computationalism (Fodor) or symbolic processing. For this section I have profited much from D. Andler’s article in Cahier du CREA N° 9.
For more on this see J. Searle, Intentionality, Cambridge U. P., 1983.
R. Shepard and J. Metzler, Science 171, 701-703 (1971).
S. Kosslyn, PsychoL Rev. 88, 46-66, 1981.
See Beh. Brain Sci. 2, 535-581 (1979).
This is the opening line of a popular textbook in neuroscience: “The brain is an unresting assembly of cells that continually receives information, elaborates and perceives it, and makes decision.” S. Kuffler and J. Nichols, From Neuron to Brain, Sinauer Associates, Boston, 2nd ed., 1984, p. 3.
D. Hubel and T. Wiesel, J. Physiol. 160, 106 (1962). For a recent account of this work see Kuffler and Nichols, op. cit. Ch. 2-4.
H. Barlow, Perception 1, 371-392.
F. Rosenblatt, Principles of Neurodynamics: Perceptrons and the Theory of Brain Mechanisms, Spartan Book, 1962.
For more on the complex early origins of self-organization ideas see I. Stengers, Cahier du CREA N° 8, pp. 7-105.
‘The logical geography of computational approaches’, MIT Sloan Conference, 1984.
For extensive discussion on this point of view see P. Dumouchel and J.-P. Dupuy (Eds.), L’Auto-organisation: De la physique au politique, Eds. du Seuil, Paris, 1983.
See for example H. von Foerster (Ed.), Principles of Self-Organization, Pergamon Press, 1962.
An accessible introduction to the modern theory of dynamical systems is: R. Abraham and C. Shaw, Dynamics: The Geometry of Behavior, Aerial Press, Santa Cruz, 3 vols., 1985.
G. Horn and R. Hill, Nature 221, 185-187 (1974).
M. Fishman and C. Michael, Vision Res., 13, 1415 (1973) and F. Morell, Nature 238, 44-46 (1972).
J. Allman, F. Miezen and E. McGuiness, Ann. Rev. Neuroscien. 8, 407-430 (1985).
F. Varela and W. Singer, Exp. Brain Res. 66, 10-20 (1987).
An interesting collection of examples is: G. Palm and A. Aersten (Eds.), Brain Theory, Springer Verlag, 1986.
The name is proposed in: J. Feldman and D. Ballard, ‘Connectionist models and their properties’, Cognitive Science 6, 205-254 (1982). For extensive discussion of current work in this direction see: D. Rumelhart and J. McClelland (Eds.), Parallel Distributed Processing: Studies on the Microstructure of Cognition, MIT Press, 1986, 2 vols.
The main idea is due to J. Hopfield, Proc. Natl. Acad. Sci. (U.S.A.), 79, 25542556 (1982).
There are many variants associated to these ideas. See in particular: G. Hinton, T. Sejnowsky, and D. Ackley, Cognitive Science 9, 147-163 (1984), and G. Toulousse, S. Dehaene, and J. Changeaux, Proc. Natl. Acad. Sci. (U.S.A.), 83, 1695-1698 (1986).
The idea is due to D. Rumelhart, G. Hinton, and R. Williams, in: Rumelhart and McClelland, op. cit., Ch. 8.
T. Sejnowski and C. Rosenbaum, ‘NetTalk: A parallel network that learns to read aloud’, TR JHU/EECS-86/01, John Hopkins Univ.
For the distinction between symbolic and emergent description and explanation in biological systems see F. Varela, Principles of Biological Autonomy, North Holland, New York, 1979, Ch. 7, and more recently S. Oyama, The Ontogeny of Information, Cambridge U. Press, 1985.
See D. Hillis, intelligence as emergent behavior, Daedalus, Winter 1989, and P. Smolesnky, ‘On the proper treatment of connectionism’, Beh. Brain Sci. 11: 1, 1989. In a very different vein J. Feldman, Neural representation of conceptual knowledge’, U. Rochester TR189 (1986) proposes a middle ground between ‘punctuate’ and distributed systems.
P. Smolesnky in: Rumelhart and McClelland, op. cit., Ch. 6.
This is extensively argued by two noted spokesmen of cognitivism: J. Fodor and S. Pylyshyn, ‘Connectionism and cognitive architecture: A critical review’, Cognition, 1989. For the opposite philosophical position in favor of connectionism see: H. Dreyfus, ‘Making a mind vs. modeling the brain: AI again at the cross-riads’. Daedalus, Winter, 1989.
Most influential in this respect is the work of H. G. Gadamer, Truth and Method, Seabury Press, 1975. For a clear introduction to hermeneutics see Palmer, Hermeneutics, Northwestern Univ. Press, 1979. The formulation of this section owes a great deal to the influence of F. Flores, see: T. Winnograd and F. Flores Understanding Computers and Cognition: A New Foundation for Design, Ablex, New Jersey, 1986.
The name is far from being an established one. I suggest it here for pedagogical reasons, until a better one is proposed.
H. Dreyfus and S. Dreyfus, Mind over Machine, Free Press/Macmillan, New York, 1986.
For this explicit way of constructing biologically inspired networks see T. Poggio, V. Torre and C. Koch, Nature 317, 314-319 (1986).
For an interesting sample of discussion in AI about these themes see the multiple review of Winnograd and Flores’s book, in Artif. Intell. (1987).
The main reference points we have in mind here are (in their English versions): M. Heidegger, Being and Time, Harper and Row, 1977; M. Merleau-Ponty, The Phenomenology of Perception, Routledge and Kegan Paul, 1962; Michel Foucault, Discipline and Punish: The birth of the prison, Vintage/Random House, 1979.
See for instance P. Watzlawick (Ed.), The Invented Reality: Essays on Constructivism, Norton, New York, 1985.
Most clearly seen in the Vienna school of Konrad Lorenz, as expressed, for example, in Behind the Mirror, Harper and Row, 1979.
See for instance E. Land, Proc. Natl. Acad. Sci. (U.S.A.) 80, 5163-5169 (1983).
P. Gouras and E. Zenner, Progr. Sensory Physiol. 1, 139-179 (1981).
F. Varela et al., Arch. Biol. Med. Exp, 16, 291-303 (1983); E. Thompson, A. Palacios, F. Varela, Beh. Brain Sci. (in press), 1992.
W. Freeman, Mass Action in the Nervous System, Academic Press, 1975.
W. Freeman and C. Skarda, Brain Res. Reviews, 10, 145-175 (1985). Significantly, a section of this article is entitled: ‘A retraction on “representation”’ (p. 169).
This biologically inspired re-interpretation of cognition was presented in H. Maturana and F. Varela, Autopoiesis and Cognition: The realization of the Living, D. Reidel, Boston, 1980, and F. Varela, Principles of Biological Autonomy, op. cit. For an introductory exposition to this point of view and more recent developments see H. Maturana and F. Varela, The Tree of Knowledge: the Biological Roots of Human Understanding, New Science Library, Boston 1987. The links with language and AI are discussed in Winnograd and Flores, op. cit.
See J. H. Holland, ‘Escaping brittleness’, in: Machine Intelligence, Vol. 2 (1986).
An interesting recent collections of diverse papers in this direction can be found in: Evolution, Games and Learning: Models for Adaptation in Machines and Nature, Physica 22D (1986). Surely, many of the contributors would not agree with our readings of their work. For an explicit example see: F. Varela, ‘Structural coupling and the origin of meaning in a simple cellular automata’, in E. Secarz, (Ed.), The Semiotics of Cellular Communications, Springer-Verlag, New York, 1987.
P. Smolesnky, op. cit., p. 260.
It is worth noting that similar arguments can be applied to evolutionary thinking today. For the parallels between cognitive representationism and evolutionary adaptationism, see F. Varela, in: P. Livingston (Ed.), op. cit. and the Introduction in this volume.
See also the remarks by Roger Schank in Al Magazine, pp. 122-135 (Fall 1985).
This is the trend within the new field of ‘Artificial Life’; see e.g. Ch. Langton (Ed.), Artificial Life, Addison-Wesley, New Jersey, 1990.